Hacking Film: What Exactly Does ‘Computational Filmmaking’ Mean?
Your smartphone has more computational power than the NASA supercomputers of the 1980s. You knew that. But did you also know that your phone has more filmmaking technology than Spielberg had 30 years ago? What’s more, advances in Machine Learning and Computational Photography are poised to deliver even more filmmaking features to smartphones than were imaginable even just a few short years ago.
Recent advances in Computational Photography (eg, synthesizing a single photo from multiple images) and a machine learning technique called a “Generative Adversarial Network” (GAN) have set the stage for an entirely new kind of filmmaking—filmmaking that is equal parts photography and digital image creation, something I’d label “Computational Filmmaking”.
In Computational Filmmaking, the line between what’s photographed and what’s digitally generated is blurred so much that the division ceases to be relevant. While it’s true that digital effects in all levels of filmmaking—including independent low budget film—are nothing new, there’s always been a distinct phase wherein live-action elements are photographed, and another phase where digital effects are added. But in the Computational Filmmaking era, all of this happens at once: the discrete phases of production, post-production and exhibition collapse into whatever is happening on your screen right this moment.
So where are we now, and where are we headed?
RIGHT NOW
While most of the really interesting developments in CP and GANs exist as “just around the corner” proof-of-concepts, there’s already a great representational implementation of these technologies happening right now on the world’s most popular camera: the iPhone.
The iPhone has been doing CP for a long while now with its HDR (High Dynamic Range) camera function—that’s when your camera takes three, rapid fire images bracketed at different exposure levels and merges them into a single image displaying a wider exposure range (latitude) than is capable within a single exposure.
On their flagship iPhone 7, Apple added a second camera with a longer lens. This second camera can be used to zoom in. Or it can be used for a Computational Photography technique Apple calls “Portrait Mode”. This mode uses both cameras, along with some very smart and fast software, to generate a “depth map” of an image. This depth map is then used to selectively blur certain pixels in the image to simulate the shallow depth of field only possible on a larger sensor with a bigger, more expensive lens. It lets the iPhone do the thing that your DSLR does, without having to kludge together a bunch of hardware to do it.
I’d go into a deep geek dive on how this is possible, but I don’t have to. My fellow Bay Area film tech comrade Stu Maschwitz has written a far better explanation than I ever could.
RIGHT AROUND THE CORNER
Adobe SkyReplace is one of those technology demonstrations that show what kind of post-production jobs artificial intelligence will eventually automate. Sky replacement is the sort of invisible, bread-and-butter visual effects gig that’s been done by thousands of artists on every single print ad, film, commercial and TV show you’ve ever seen. No one notices it, but in commercial media the sky always looks nice. But the reality is that someone replaced a dull sky with a spectacular one, frame by frame, by hand.
SkyReplace is poised to automate this chore using Machine Learning, and backed up by Adobe, a company that has a vast repository of stock art sky images to substitute into your images. For that matter, Adobe has stock art for everything. Don’t like the couch in the shot? Replace it with a different one. The sky is not the limit.
THE TRULY WEIRD
A Generative Adversarial Networks (GAN) is a statistical probability model that continuously tries to fool other models, until it can do so with ease. When it finally succeeds, the GAN can generate realistic looking images. Meaning, if you show one of these neural networks a million cat images, it can do a pretty good job of recognizing a cat when it sees one. Further, it can generate original cat images all on its own. Which is good—the internet needs more cats. Watch them be created here:
The more images a GAN sees, the better it gets at identifying specific objects in new images. This leads to really cool things like searching untagged pictures just by typing in a sentence, like “a blue bird sitting on a fence”.
Something else—and at least for me, this is the truly strange thing that these neural networks can do—is generate original images. It’s still early and the images are small, but take a look at this block of images:
…they were all generated by a GAN. After the network had been trained to look at flowers, it was able to start generating its own flower-like imagery. So how long until a photorealistic image can be generated just by typing a sentence? Or writing a script?
Not any time soon, so don’t get too excited about this—yet. For a better take on exactly how a GAN works, take a look at this video:
STATE OF THE ART
While text-to-picture tools are in their earliest stages, Adobe and DeepMind both have neural network systems that can produce text-to-speech that is indistinguishable from actual human voices. DeepMind’s WaveNet can create human sounding voices. It can also “listen” to terabytes of music and then start composing its own, original scores.
Adobe’s “Voco” promises something even more realistic voice generation by using someone’s actual voice.
This so called “Photoshop for audio” is a demo at this point, but will most likely get rolled into some future version of Creative Cloud. How long before you’ll be able to buy the “celebrity voice pack” license and have Morgan Freeman narrating your home movies?
CONCLUSION
Advances in Computational Photography and Machine Learning are already here, and they will transform how we create images in ways that are hard to imagine right now. But I’ll still take a stab at it:
In a few years, when you want to make a movie, you’ll be able to pick up your phone and tell it, via a natural language processor, what you want your movie to look like. This may be specific like “Arri Alexa 2011” or “Anamorphic 35mm, 1970’s”. Or you’ll say a mood, or a movie title.
Your phone, now sporting a half a dozen lenses and impossibly fast image processors, linked to an unlimited number of GPUs in nVidia’s cloud somewhere will return the result. Your screen will be a real-time director’s viewfinder with all the optical artifacts, lens flares, color profiles and depth-of-field cues of your chosen system. Further, SkyReplace will offer you up some options for your exterior shot that more matches the style of your selection.
The real world that you’re putting in front of the camera(s) is merely a starting point, something you pile an amalgam of digitally generated, live action and stock images on top of. If your actor misses a line, or there’s too much ambient noise it won’t matter, Voco has already gathered enough dialogue to do an auto ADR based on your script. Music? WaveNet has already generated your score in the style of John Adams.
The punchline: when everyone can do this, everyone will do this. Think about how amazing Prisma was when it released last Summer. Remember all those cool auto-art images suddenly clogging your Instagram feed?
Prisma is a great example of Machine-Learning-on-smartphone image processing for everyone. It does a better job at converting photos to “art images” than anything that has come before it. It does a better job than almost every person could do armed with Photoshop and a deadline, and it does it for free and in a few seconds. Suddenly this becomes the value of the art, even when it looks great.
And when was the last time you used that cool “Mononoke” filter?
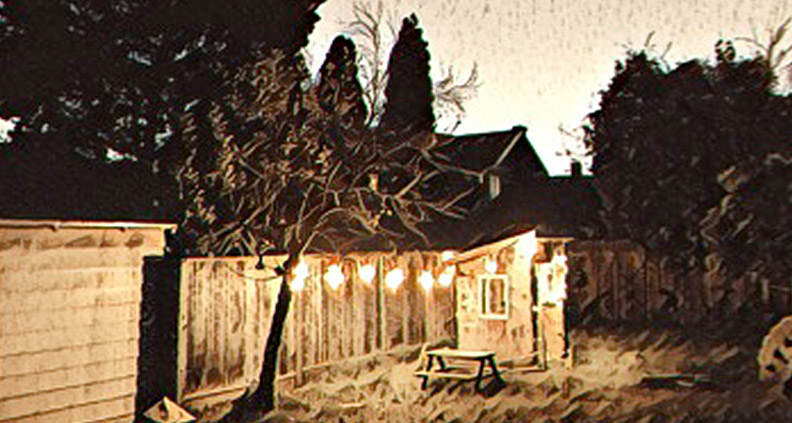
To learn more about the convergence of art, science and filmmaking, check out more of Eric Escobar’s Hacking Film series.
To learn more about Film Independent, subscribe to our YouTube channel. And be our friend on Twitter, Instagram and Facebook. To learn how to become a Member of Film Independent just click here.